Big O Complexity Cheat Sheet
- O N Cheat Sheet
- Big O Cheat Sheet Pdf
- Big O Time Complexity Cheat Sheet
- Big 0 Cheat Sheet
- Big-o Algorithm Complexity Cheat Sheet Pdf
About: I made this website as a fun project to help me understand better: algorithms, data structures and big O notation. And also to have some practice in: Java, JavaScript, CSS, HTML and Responsive Web Design (RWD). Big-O Algorithm Complexity Cheat Sheet Created Date: 7/10/2016 7:49:20 PM. Jan 16, 2020 The average case complexity describes the expected performance of the algorithm. Sometimes involves calculating the probability of each scenarios. It can get complicated to go into the details and therefore not discussed in this article. Below is a cheat-sheet on the time and space complexity of typical algorithms. Big O Cheatsheet for Common. Algorithm taking Omega (n log n) takes at least n log n time but has no upper limit. An algorithm taking. Theta (n log n) is far preferential since it takes AT LEAST n log n (Omega n log n) and NO MORE THAN. N log n (Big O n log n). Big-O Algorithm Complexity Cheat Sheet http://bigocheatsheet.com/. View Big-O Algorithm Complexity Cheat Sheet.pdf from COMPUTER S 101 at Baruch College, CUNY. Download PDF Big-O Cheat Sheet SHARES Share Tweet Share Share Submit Know Thy Complexities!
Recently I signed up for Algorithms: Design and Analysis course. Most of the time Test Automation doesn't require to know which algorithm sorts best, but I still believe that knowing that a good programmer is not the one who knows a lot of languages, but the one knows algorithms well and which one to apply where.
One of the tricky parts for me was to understand the 'complexity' of each algorithm. Usually, it's stated as O(n), O(1) etc, which wasn't really clear for me. But now it all became easy thanks to Complexity Cheat Sheet . Import gmail contacts to iphone. This page is all about algorithms complexity given in very clear and understandable form. Check it out :
When measuring the efficiency of an algorithm, we usually take into account the time and space complexity. In this article, we will glimpse those factors on some sorting algorithms and data structures, also we take a look at the growth rate of those operations.
O N Cheat Sheet
Big-O Complexity Chart
First, we consider the growth rate of some familiar operations, based on this chart, we can visualize the difference of an algorithm with O(1) when compared with O(n2). As the input larger and larger, the growth rate of some operations stays steady, but some grow further as a straight line, some operations in the rest part grow as exponential, quadratic, factorial.
Sorting Algorithms
Big O Cheat Sheet Pdf
In order to have a good comparison between different algorithms we can compare based on the resources it uses: how much time it needs to complete, how much memory it uses to solve a problem or how many operations it must do in order to solve the problem:
- Time efficiency: a measure of the amount of time an algorithm takes to solve a problem.
- Space efficiency: a measure of the amount of memory an algorithm needs to solve a problem.
- Complexity theory: a study of algorithm performance based on cost functions of statement counts.
Sorting Algorithms | Space Complexity | Time Complexity | ||
Worst case | Best case | Average case | Worst case | |
Bubble Sort | O(1) | O(n) | O(n2) | O(n2) |
Heapsort | O(1) | O(n log n) | O(n log n) | O(n log n) |
Insertion Sort | O(1) | O(n) | O(n2) | O(n2) |
Mergesort | O(n) | O(n log n) | O(n log n) | O(n log n) |
Quicksort | O(log n) | O(n log n) | O(n log n) | O(n log n) |
Selection Sort | O(1) | O(n2) | O(n2) | O(n2) |
ShellSort | O(1) | O(n) | O(n log n2) | O(n log n2) |
Smooth Sort | O(1) | O(n) | O(n log n) | O(n log n) |
Tree Sort | O(n) | O(n log n) | O(n log n) | O(n2) |
Counting Sort | O(k) | O(n + k) | O(n + k) | O(n + k) |
Cubesort | O(n) | O(n) | O(n log n) | O(n log n) |

Data Structure Operations
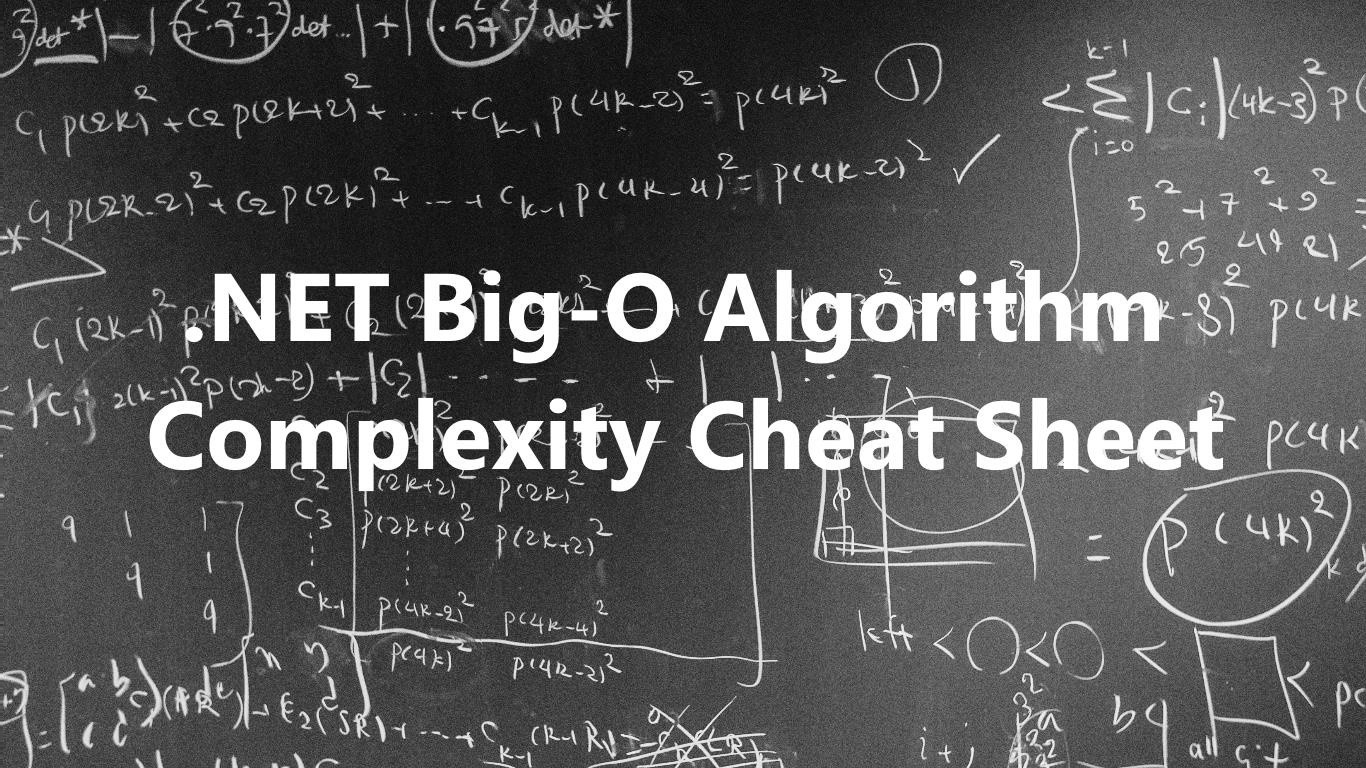
In this chart, we consult some popular data structures such as Array, Binary Tree, Linked-List with 3 operations Search, Insert and Delete.
Data Structures | Average Case | Worst Case | ||||
Search | Insert | Delete | Search | Insert | Delete | |
Array | O(n) | N/A | N/A | O(n) | N/A | N/A |
AVL Tree | O(log n) | O(log n) | O(log n) | O(log n) | O(log n) | O(log n) |
B-Tree | O(log n) | O(log n) | O(log n) | O(log n) | O(log n) | O(log n) |
Binary SearchTree | O(log n) | O(log n) | O(log n) | O(n) | O(n) | O(n) |
Doubly Linked List | O(n) | O(1) | O(1) | O(n) | O(1) | O(1) |
Hash table | O(1) | O(1) | O(1) | O(n) | O(n) | O(n) |
Linked List | O(n) | O(1) | O(1) | O(n) | O(1) | O(1) |
Red-Black tree | O(log n) | O(log n) | O(log n) | O(log n) | O(log n) | O(log n) |
Sorted Array | O(log n) | O(n) | O(n) | O(log n) | O(n) | O(n) |
Stack | O(n) | O(1) | O(1) | O(n) | O(1) | O(1) |
Growth of Functions
Big O Time Complexity Cheat Sheet

Zen life. The order of growth of the running time of an algorithm gives a simple characterization of the algorithm’s efficiency and also allows us to compare the relative performance of alternative algorithms.
Big 0 Cheat Sheet
Below we have the function n f(n)
with n as an input, and beside it we have some operations which take input n
Web todoist. and return the total time to calculate some specific inputs.

Big-o Algorithm Complexity Cheat Sheet Pdf
n f(n) | log n | n | n log n | n2 | 2n | n! |
---|---|---|---|---|---|---|
10 | 0.003ns | 0.01ns | 0.033ns | 0.1ns | 1ns | 3.65ms |
20 | 0.004ns | 0.02ns | 0.086ns | 0.4ns | 1ms | 77years |
30 | 0.005ns | 0.03ns | 0.147ns | 0.9ns | 1sec | 8.4×1015yrs |
40 | 0.005ns | 0.04ns | 0.213ns | 1.6ns | 18.3min | — |
50 | 0.006ns | 0.05ns | 0.282ns | 2.5ns | 13days | — |
100 | 0.07 | 0.1ns | 0.644ns | 0.10ns | 4×1013yrs | — |
1,000 | 0.010ns | 1.00ns | 9.966ns | 1ms | — | — |
10,000 | 0.013ns | 10ns | 130ns | 100ms | — | — |
100,000 | 0.017ns | 0.10ms | 1.67ms | 10sec | — | — |
1’000,000 | 0.020ns | 1ms | 19.93ms | 16.7min | — | — |
10’000,000 | 0.023ns | 0.01sec | 0.23ms | 1.16days | — | — |
100’000,000 | 0.027ns | 0.10sec | 2.66sec | 115.7days | — | — |
1,000’000,000 | 0.030ns | 1sec | 29.90sec | 31.7 years | — | — |
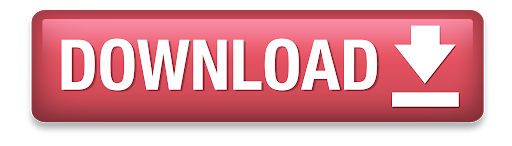